In 2017, Mike Nilsen, PT, DPT, determined that 40% of his patients with neck pain failed to progress or show clinically meaningful improvement.
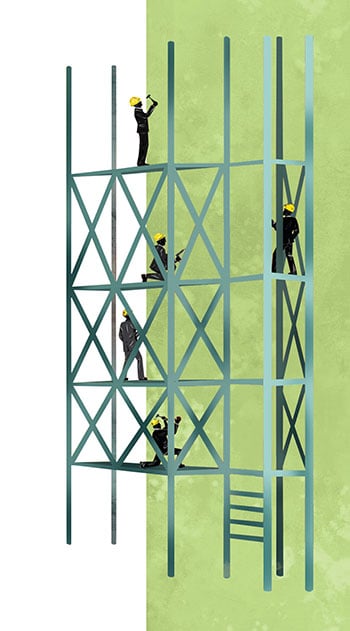
"I wasn't very happy with this," he says, "so I started performing chart reviews. I looked at the patients who didn't improve to see if I'd made any glaring mistakes. Did I not assess something? Did I not progress something?"
Nilsen, a staff physical therapist (PT) with Intermountain Healthcare in West Valley City, Utah, reviewed APTA's clinical practice guidelines and Intermountain's care process models, looking for discrepancies between patients who did and did not improve. Although he confirmed that his treatment followed protocols and best practices, he also saw that he had better results when he progressed treatment a bit further. He identified a few small tweaks that could make a big difference.